Technology
Performance Maximizer
Performance Maximizers derive high-value user rankings that are likely to achieve advertisers' KPIs and the advertiser KPI indicator is maximized by allocating advertisements based on the derived rankings.
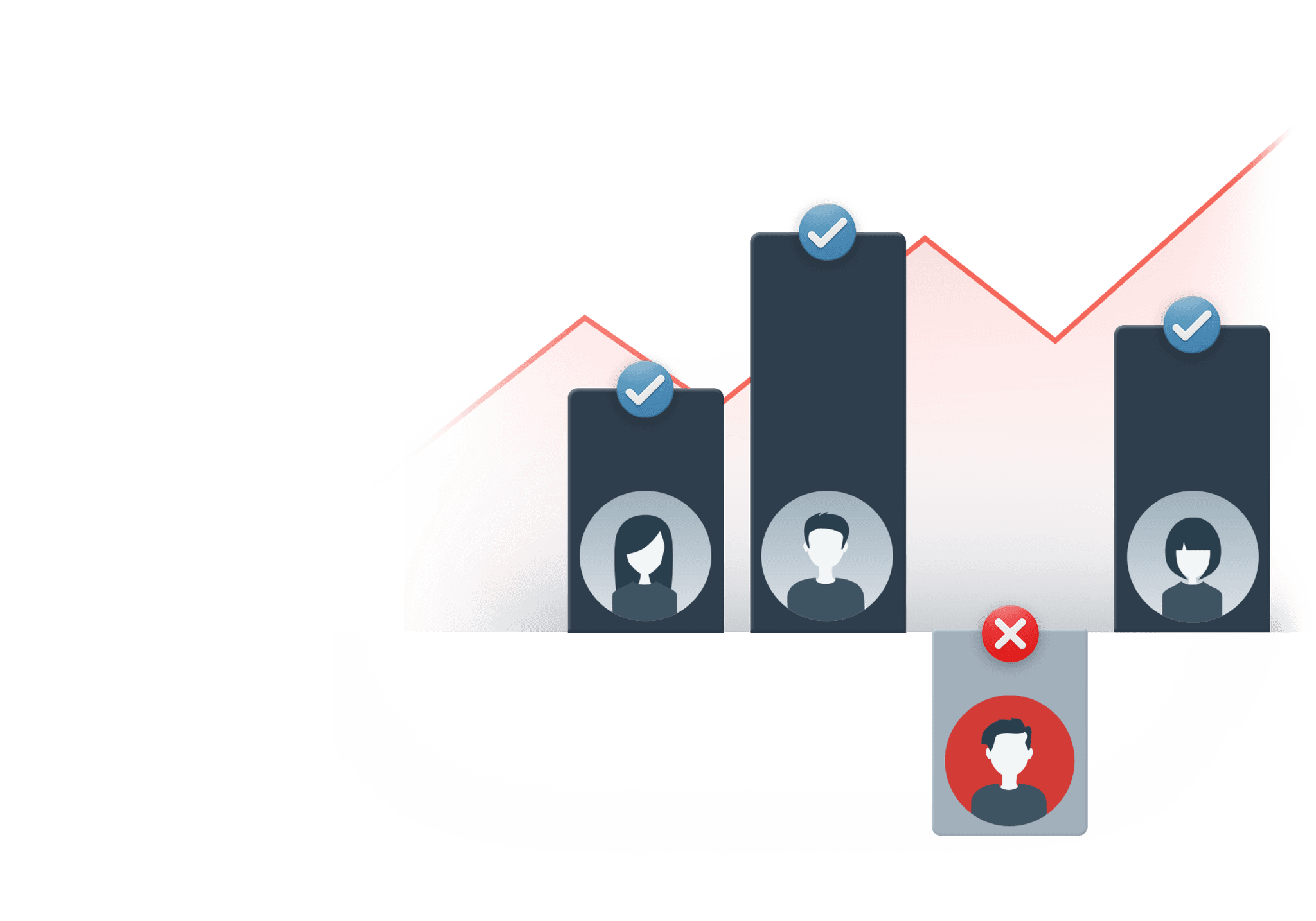
Ad exposure rankings based on inferred user value
Performance Maximizer predicts user engagement in KPI actions and adjusts ad exposure rankings accordingly, based on learned user activity data. Performance Maximizer selectively targets users for ad exposure, utilizing relevant learning data to enhance campaign performance, focusing on objectives like user acquisition and maximizing purchases. Leveraging a neural network model(NNM) and Buzzvil's vast data from millions of users, Performance Maximizer optimizes advertiser performance by accurately anticipating user behavior.
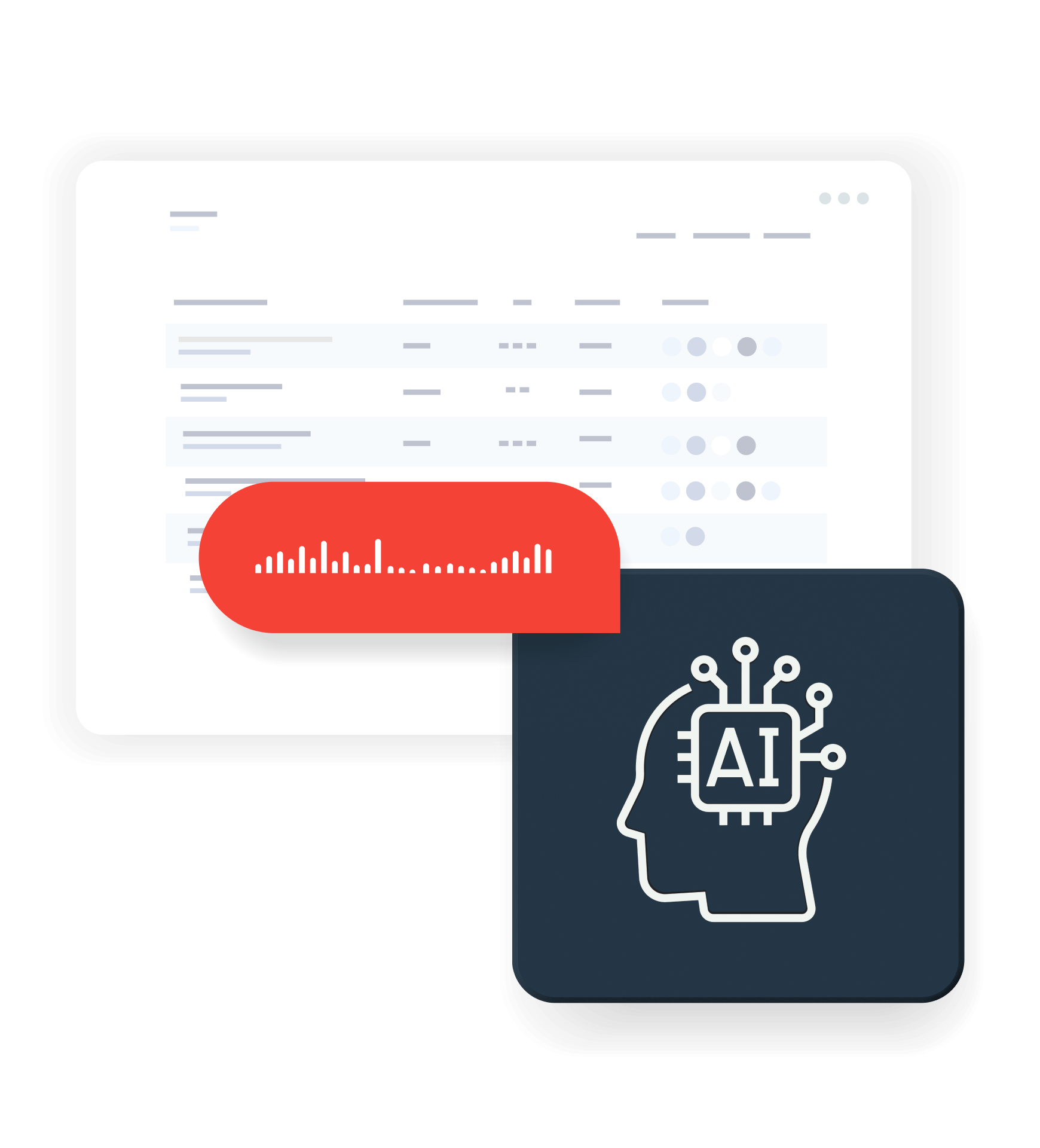
(1) UA Maximizer
Predicting the possibility of UA, UA Maximizer
How can we find customers who will actually become customers of our service, not just receive rewards and then churn? UA Maximizer apply a combination of a Seed Amplification model learning from users' ad data and a Similarity Inference model learning from Buzzvil's dataset of over 600 million entries to identify the most suitable users for ad allocation through user similarity comparison.
Generate user groups similar to a small test user set of user characteristics.
Identify user patterns and generate target groups with similar patterns by analyzing data of users acquired during the campaign.
Determining ad request user rankings according to the similarity among users.
Leveraging Buzzvil's dataset of over 600 million entries, UA Maximizer analyze the similarity between existing users and ad request users to assign user value rankings.
Campaign performance prediction through pre-campaign simulation, allocation, and execution system
Predicting ad engagement rates in advance through offline inference performance analysis targeting top-ranked users, ads are selectively allocated to users based on these predictions. Performance is continually improved by regularly updating targeting.
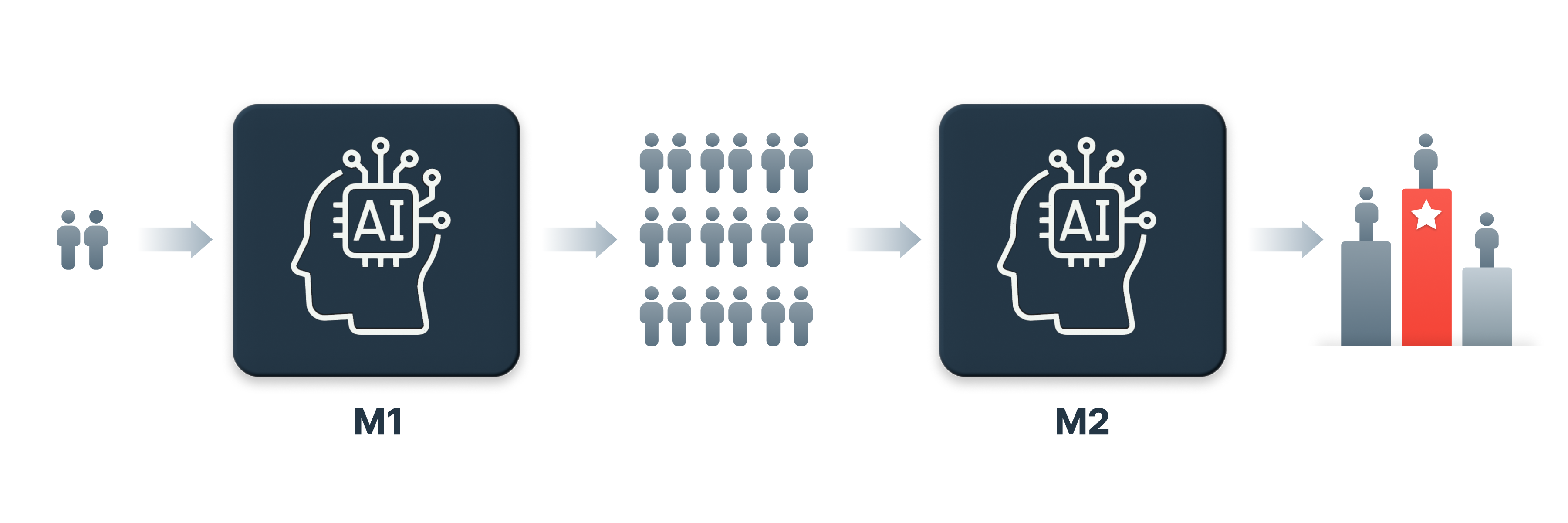
(1) UA Maximizer
Predicting the possibility of UA, UA Maximizer
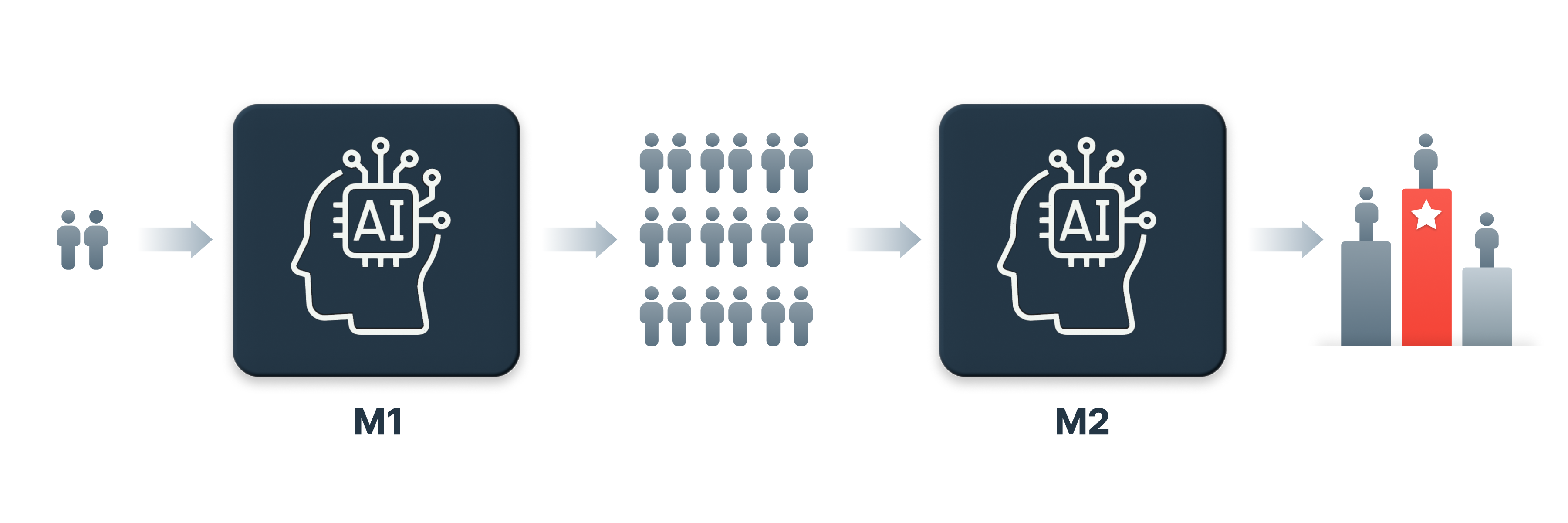
How can we find customers who will actually become customers of our service, not just receive rewards and then churn? UA Maximizer apply a combination of a Seed Amplification model learning from users' ad data and a Similarity Inference model learning from Buzzvil's dataset of over 600 million entries to identify the most suitable users for ad allocation through user similarity comparison.
Generate user groups similar to a small test user set of user characteristics.
Identify user patterns and generate target groups with similar patterns by analyzing data of users acquired during the campaign.
Determining ad request user rankings according to the similarity among users.
Leveraging Buzzvil's dataset of over 600 million entries, UA Maximizer analyze the similarity between existing users and ad request users to assign user value rankings.
Campaign performance prediction through pre-campaign simulation, allocation, and execution system
Predicting ad engagement rates in advance through offline inference performance analysis targeting top-ranked users, ads are selectively allocated to users based on these predictions. Performance is continually improved by regularly updating targeting.
(2) GMV Maximizer
Maximizing purchase conversions, GMV Maximizer
GMV Maximizer maximizes purchase performance in ad campaigns by identifying users likely to make purchases on e-commerce. It learns from both user and e-commerce data to infer the probability of a specific user triggering a purchase on a particular platform.
Specialized data learning at the platform level to account for differences across e-commerce platforms
User data is separated by platform to focus on learning, considering slight variations in user activity across different platforms.
Accurate purchase probability prediction through learning from data within e-commerce platforms.
Reflecting various behavioral data, including users' actual purchase data, the model predicts the probability of users exposed to ads actually purchasing the product, thereby assigning ad exposure rankings.
A campaign performance prediction, allocation, and execution system through pre-campaign simulation.
Running campaign simulations targeting top-ranked users to predict ad engagement rates in advance, then allocating ads to test users based on these predictions.
E-commerce-specific purchase probability prediction performance
Platform C
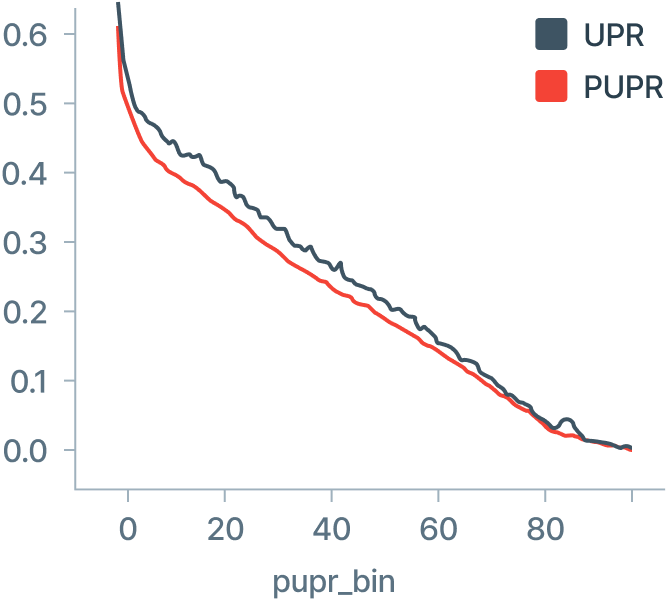
Platform E
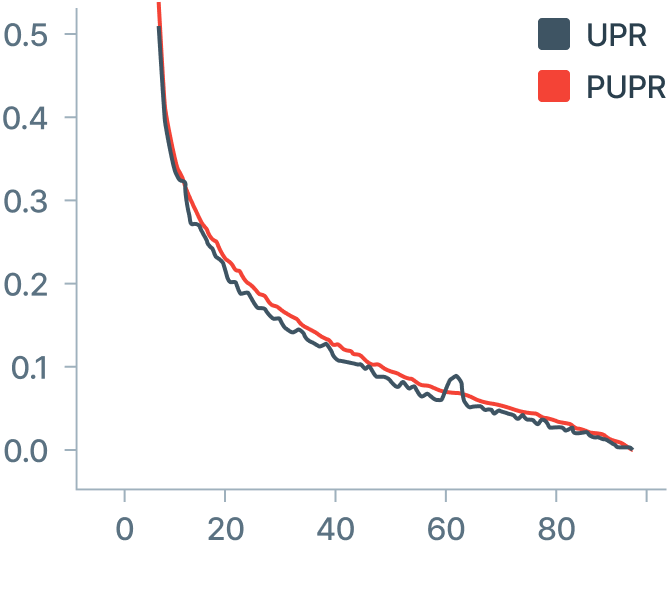
Platform W
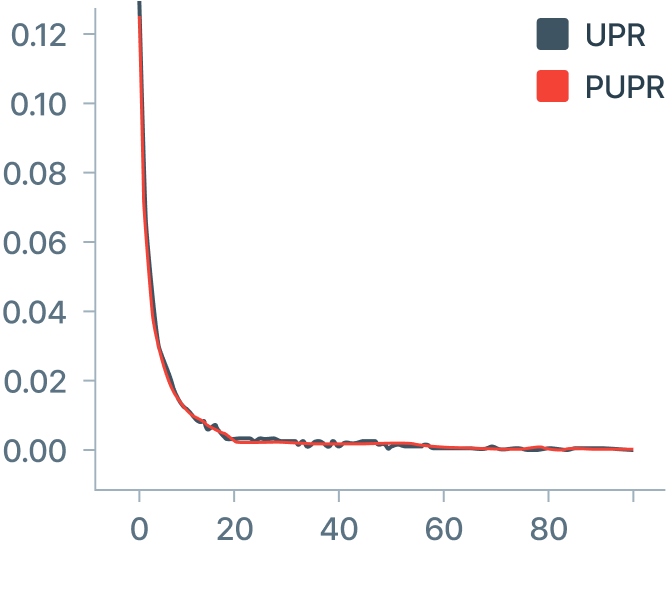
(2) GMV Maximizer
Maximizing purchase conversions, GMV Maximizer
GMV Maximizer maximizes purchase performance in ad campaigns by identifying users likely to make purchases on e-commerce. It learns from both user and e-commerce data to infer the probability of a specific user triggering a purchase on a particular platform.
Specialized data learning at the platform level to account for differences across e-commerce platforms
User data is separated by platform to focus on learning, considering slight variations in user activity across different platforms.
Accurate purchase probability prediction through learning from data within e-commerce platforms.
Reflecting various behavioral data, including users' actual purchase data, the model predicts the probability of users exposed to ads actually purchasing the product, thereby assigning ad exposure rankings.
A campaign performance prediction, allocation, and execution system through pre-campaign simulation.
Running campaign simulations targeting top-ranked users to predict ad engagement rates in advance, then allocating ads to test users based on these predictions.
E-commerce-specific purchase probability prediction performance
Platform C
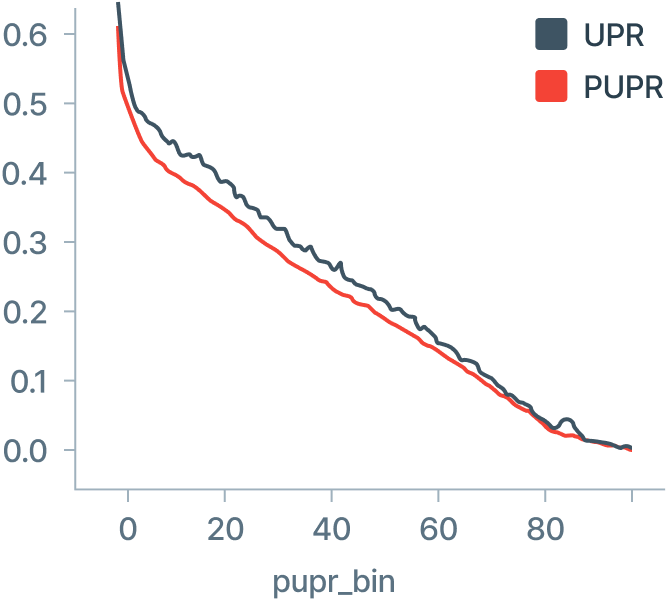
Platform E
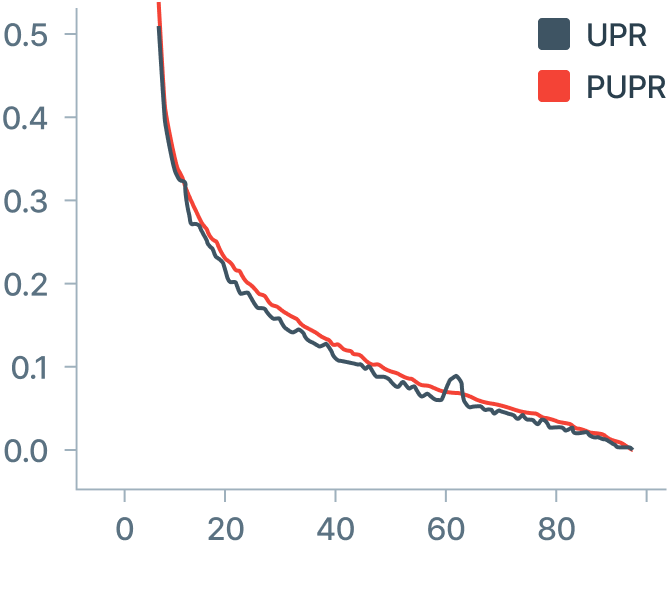
Platform W
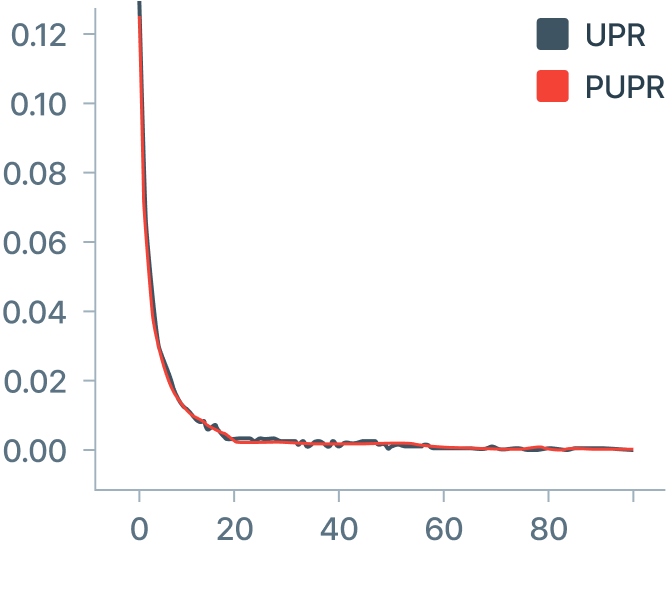
Buzzvil’s core technology
Dynamic Rewards: The key to accelerating purchase journeys
Where Ad meets innovation, Buzzvil
Experience comprehensive brand growth with Buzzvil's three product lines, which cover advertising, marketing, and app monetization
Advertise
High-Performing Reward Ads
Achieve marketer's KPI quickly with reward ads.
Activate
Point-based CRM Marketing
Acquire and activate customers with CRM marketing solution
Monetize
Monetization for App Growth
Create ad inventories and generate a new revenue stream.
Innovation backed by tangible results
Real-time data processing capacity
50K TPS
Daily data production
3TB+
Number of partners
400+
MAU
20 million +